Key Takeaways from Accelerate: How Financial Services and Manufacturing Companies Leverage Data and AI for Measurable ROI
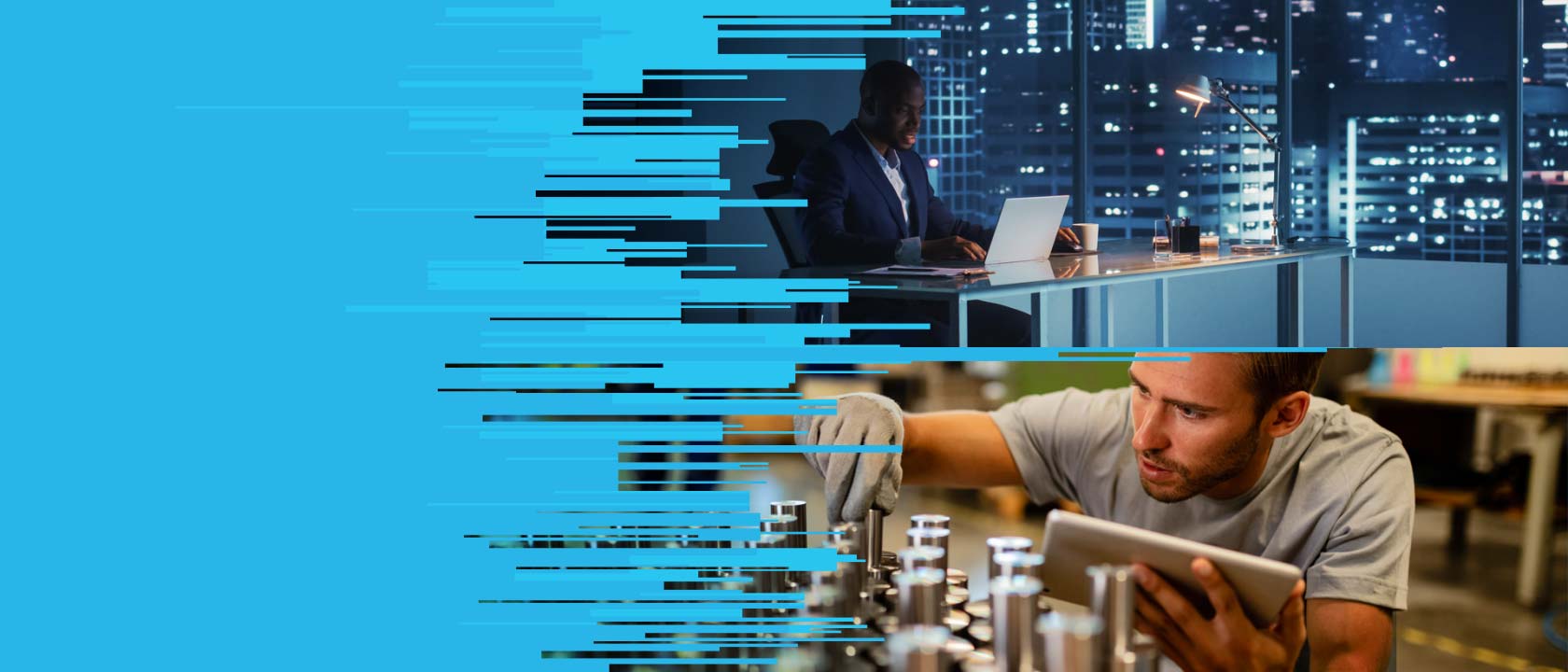
For many organizations across industries, the era of experimental AI has given way to the era of practical implementation. Even those companies still testing and evaluating AI solutions are shifting away from the art of the possible to focus more closely on what will soon produce measurable ROI.
“It will no longer be enough for your organization to merely use AI to win the approval of company leadership,” says Samuel Lee, Product Marketing Director for Financial Services at Snowflake. “Instead, organizations must actually be driving value from their AI implementations, and leaders will face increased pressure to quantify their AI investments and the wider business impact.”
That was a key message in both of Snowflake’s most recent industry-focused events, Accelerate Financial Services and Accelerate Manufacturing, which took place in March. The events, in collaboration with Snowflake partner Amazon Web Services (AWS), were a valuable opportunity for professionals and leaders to learn how to overcome data and AI implementation obstacles, scale AI with confidence and achieve successful business outcomes.
Sessions spotlighted the latest industry trends, innovative use cases and strategies for creating a winning enterprise data and AI strategy. Snowflake experts, customers and leaders from AWS provided strategic insight and practical tips for optimizing AI strategy, demos for key use cases and best practices.
Here are seven more insightful takeaways:
A data cloud strategy is the first step for companies looking to use AI in their operations. “[Leveraging] advanced AI/ML almost always starts with getting all your data into the cloud in an organized, systematic manner,” says Magnus Akesson, Worldwide Head of Industrial Vertical Solutions GTM at AWS.
The accessibility of advanced AI capabilities is transforming and streamlining operational tasks. "We are definitely seeing more operational efficiency features, especially in tasks like document processing,” says Debanit Das, Senior Director of Technology at Northwestern Mutual. “Previously, processing unstructured data was time-consuming and painful, requiring manual work. Now, automating these tasks using LLM and ML capabilities allows us to tap into them more cost effectively. While implementing semantic analysis and pattern recognition models was always possible, it was costly to have a dedicated research and development team. Now, with a little prompt engineering, you can access those capabilities."
Regulatory compliance is top of mind as leaders adopt emerging technologies. “Many financial services companies are leveraging AI — specifically, generative AI and agentic automation,” says Lorraine Knerr, Global Head of Gen AI and Data Solutions Strategy and Architecture at AWS. “They want to know that they’re ready for the intense regulatory scrutiny that comes with that, especially in terms of data privacy.”
As AI models become increasingly sophisticated, a shift toward comprehensive governance is emerging. “There's always been model governance, but there's now a lens of governing not only the data that is going into training AI models, but also governing the responses that come out of the model,” says Awah Teh, VP of Data Governance and Privacy Engineering at Capital One.
Leading companies have been implementing multifaceted strategies to adapt as supply chains undergo significant transformations. These include “embracing digital transformation, fostering more collaborative supplier relationships, implementing agile and lean manufacturing and developing resilient and flexible networks,” says Saurabh Vijayvergia, AI Strategy and Engineering Leader at Deloitte. “One of the most significant emphases for organizations continues to be data collaboration to enhance efficiency and integration across the end-to-end value chain. … By sharing data seamlessly across the value chain, these organizations can achieve enhanced visibility and insights into every facet of their supply chain, which enables proactive identification and mitigation of potential disruption.”
Before applying new technologies, manufacturers should make sure their data is in order. “Technology tools are great. However, look into [improving] processes first. … Set up a data governance process and master data management processes that inventory all the data that you have,” says Ramin Rastin, Senior Vice President of Data Engineering and Advanced Data Sciences (AI/ML) at GXO Logistics. “Correct all of those processes first, because once you launch, say, a global data lake with a partner like Snowflake, it makes onboarding that tool much easier, much faster and a lot more agile. And what you get out of that platform with AI tools becomes so much more meaningful.”
Gen AI is already reshaping manufacturing supply chains. “Generative AI is being used all over manufacturing organizations, whether it’s in planning to improve demand forecasting, production processes or even reducing inventory,” says Tim Long, Manufacturing Global Industry GTM Lead at Snowflake. “Across the manufacturing floor, gen AI is emerging as a leading technique in helping to identify failures and root causes.” Also, Long says, “gen AI is enabling cognitive supply chains, helping ensure the product is delivered as efficiently as possible to the end customer.”
Couldn’t make it? You can still catch the events on demand: Accelerate Financial Services and Accelerate Manufacturing.