AI-Driven ABM: Scaling Precision and Impact for B2B Growth
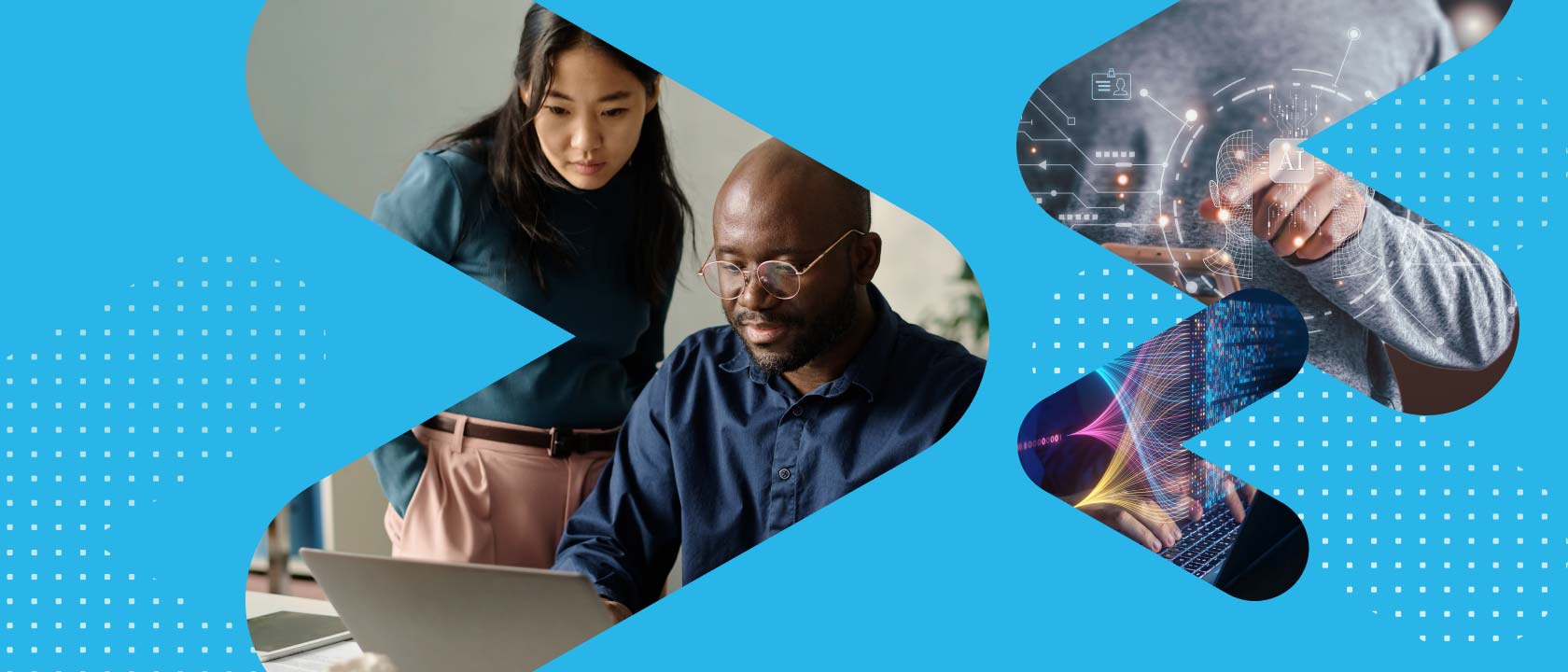
Discover how Snowflake's ABM team achieved a 2.3x lift in meetings booked and a 54% increase in CTR by using Snowflake AI for targeted campaigns and more personalized messaging — while optimizing both budget and engagement.
We’ve seen how Snowflake AI tools are transforming outcomes for our customers. From saving 4,000 hours a year on manual email intake to treating more patients in emergency rooms to saving 75% of costs, AI in Snowflake is making a real impact on businesses around the world.
That same transformative power is at work within Snowflake, too. Here, we dive into how Snowflake’s account-based marketing (ABM) team is using Snowflake AI tools to drive significant improvements in how we allocate resources and connect with high-value accounts.
Precision targeting without breaking the bank
Like many enterprise marketing teams, the ABM team faced a common dilemma: how to optimally distribute advertising budget across different territories, account types and industries. The team needs to target the right accounts with laser-like precision but still be mindful of resources.
Previously, the Snowflake ABM team’s budget allocation was broad — divided by territory using different tools. While functional, this lacked the granular insight needed for true optimization. The team couldn't dynamically adjust spend based on key account characteristics and real-time engagement, and the team was looking for a way to achieve better precision on a budget while also helping meet the team’s quarterly business goals for booking meetings.
Developing an AI model for predictive ABM
Their solution was to build a “meeting propensity” AI model, powered by Snowflake Cortex AI, that goes beyond basic targeting. This tool doesn't just identify accounts suitable for ABM campaigns — it helps predict which ones are most likely to respond positively to outreach efforts and lead to booked meetings. With it, the ABM team saw several key advantages:
Data-driven budget allocation: ABM team members can now justify and propose budgets for each region and program, backed by concrete predictive modeling. This reduces guesswork and optimizes resource allocation.
Increased accountability and performance: Clearer predictions drive accountability and a focus on delivering measurable results.
Agile optimization: The model allows for real-time budget adjustments based on account performance and changing market dynamics — cultivating an adaptability that’s crucial for scaling companies such as Snowflake.
They put the model to the test with a straightforward hypothesis: Could they predict meeting outcomes with 80% certainty and boost meeting rates by 3% through optimized spending?
They could. “We saw a 2.3x lift in meetings booked in the high-potential accounts compared to the lower potential accounts,” says Breanna Cherman, Senior ABM Strategic Operations Lead at Snowflake. “Essentially, we found that this model can help us spend 38% less money for more engagement with our audience and more meetings in the right accounts.”
The ABM team has fully implemented the model into its sales territory planning. “By using the AI model to pinpoint high-potential accounts and accelerate our sales strategy, we're not just winning more,” Cherman says. “We're winning faster, smarter and with greater precision than ever before."
Personalizing ad copy for thousands of accounts at scale
The ABM team also teamed up with the Snowflake brand team to tailor ad messaging for top-priority sales accounts. Given the number of accounts ABM handles and how important personalized copy is for engagement, the team decided to test whether AI-generated ad copy could improve click-through rates (CTRs) and further enhance overall campaign ROI, which would help in handling scale.
Account-based marketing used Cortex AI and worked closely with Snowflake’s brand team to develop guidelines and prompts for a large-language model to execute the task, and then built a Streamlit app powered by the prompt in Cortex AI.
Method #1: AI vs. historical benchmarks
In the first approach, they compared the performance of AI-generated ad copy against historical performance data or established regional benchmarks for their campaigns. This allowed for a direct assessment of whether AI would outperform previously established best practices.The brand team conducted reviews to ensure the ad copy was meeting brand standards and writing quality. AI-generated copy performed better overall, often demonstrating improved CTRs compared to the historical or regional benchmarks.
Method #2: Head-to-head A/B testing on LinkedIn
To further validate these findings, the ABM team conducted A/B tests on LinkedIn. They ran campaigns where half the target audience saw original, human-written ad copy, while the other half saw AI-generated copy. This head-to-head comparison provided a clear and unbiased measure of the AI's effectiveness.
AI-generated copy again hit the mark. After some final tests, ultimately campaigns using the AI-generated creative saw a 54% lift in CTR compared to those using the original copy. “With AI, we no longer have to choose between efficiency and personalization — we can have both,” says Maila Ruggiero, Account-Based Marketing Manager at Snowflake. "Now we can scale messaging that cuts through the noise and engages prospects by addressing their needs, challenges and goals.”
To date, the team has successfully rolled out and implemented the model and is now focused on enhancing its capabilities to generate expanded outputs and integrate it into additional automations within the campaign execution process.
To learn more about how customers are using generative AI with Snowflake for real-world results, download the Secrets of Gen AI Success report or explore Snowflake’s AI solutions.