Introducing Arctic Agentic RAG: Smarter, Faster and More Reliable AI for Enterprise
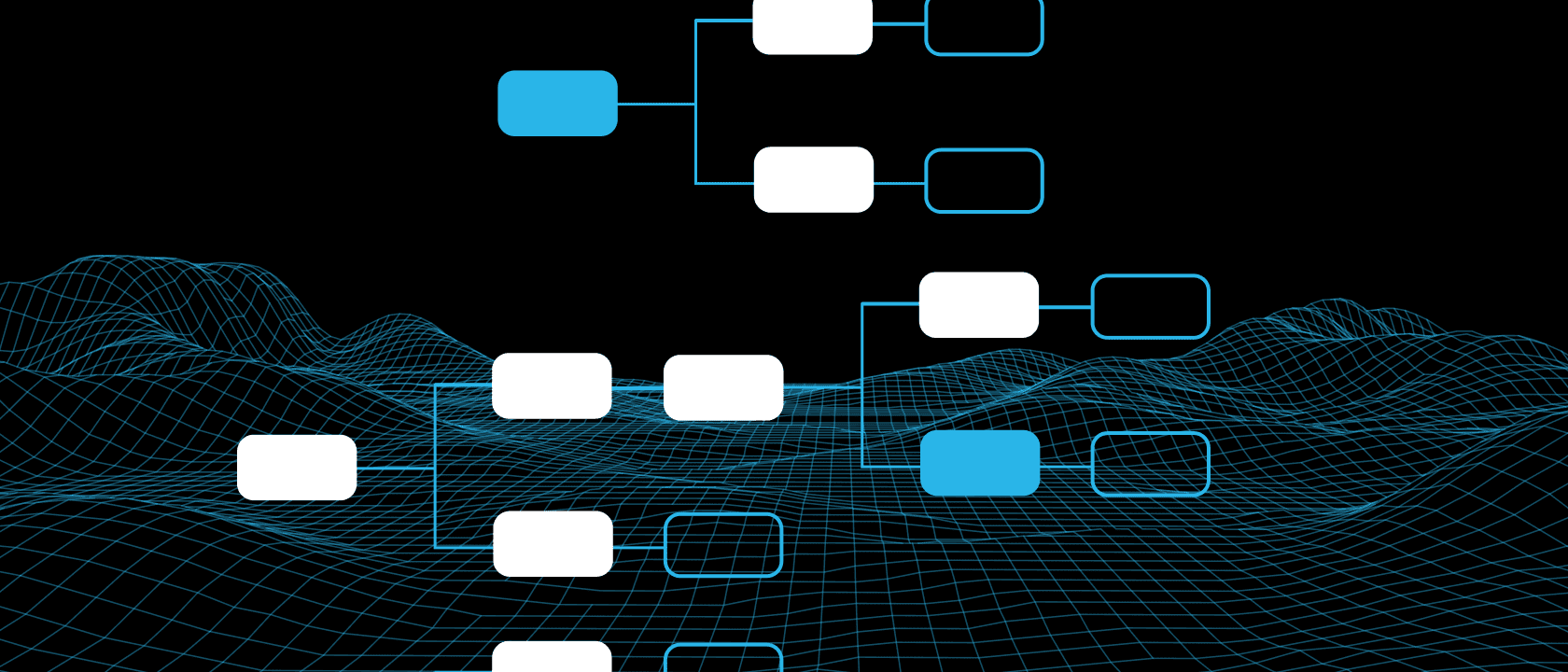
Retrieval-augmented generation (RAG) has transformed enterprise AI by enabling real-time knowledge retrieval and synthesis. By combining pretrained model knowledge with dynamic retrieval, RAG improves accuracy, adaptability and relevance across various enterprise applications:
Enterprise search: Helping employees retrieve and synthesize critical business insights.
Data analysis: Supporting analysts in extracting insights from structured and unstructured data.
Customer support: Powering AI-driven assistance with real-time, context-aware responses.
However, despite RAG’s promise, traditional RAG systems face critical challenges in enterprise environments, where accuracy, efficiency and trust are paramount. Enterprises demand more than static retrieval — they require AI that can understand, reason and adapt in real time.
This is where agentic RAG comes in — a next-generation approach that brings intelligence, adaptability and scalability to retrieval and generation. At Snowflake, we are building Arctic Agentic RAG, an innovative and enterprise-ready framework that makes AI-driven retrieval smarter, faster and more reliable than ever before.
In this blog series, we’ll explore how Arctic Agentic RAG tackles enterprise AI challenges, the innovations behind it and how we are bridging research with real-world applications and open sourcing key components.
The problem: Why traditional RAG may fall short in enterprise AI
While RAG is a powerful tool, applying it effectively in enterprise settings is complex. Here are some of the biggest challenges:
Ambiguity in queries: Business queries are often vague or underspecified. A static retrieval approach struggles to clarify intent, potentially leading to incorrect or incomplete responses.
Diverse input modalities: Enterprise data isn’t just text — it spans tables, charts, PDFs and databases. Handling multimodal queries requires more sophisticated retrieval strategies.
Complex information needs: Many enterprise AI queries require reasoning over multiple sources, merging, filtering and synthesizing insights — a challenge for traditional RAG systems.
Trust and grounding: In industries such as finance and healthcare, accuracy is nonnegotiable. RAG must not only retrieve information but verify and explain its outputs.
- Varying business priorities: Enterprise AI needs differ. Some users prioritize precision, while others value a balance of speed, accuracy and cost efficiency. A one-size-fits-all retrieval approach doesn’t work for all businesses.
The big question: How can RAG evolve to meet enterprise AI needs?
To make RAG more trustworthy, adaptive and business-ready, we need a new approach — one that moves beyond static retrieval and embraces dynamic reasoning.
The power of agentic RAG
Agentic RAG transforms traditional retrieval by introducing reasoning, iteration and decision-making into the process. Instead of retrieving and generating in a single step, agentic RAG dynamically refines retrieval and synthesis based on context.
Here’s how:
Iterative query refinement: Rather than blindly retrieving documents, an agentic system clarifies queries step by step, for more precise and user-aligned responses.
Multistep reasoning and adaptive retrieval: Instead of treating retrieval and generation as separate, rigid steps, agentic RAG reasons through queries, refines retrieval strategies and integrates results progressively.
Intelligent source selection and fusion: For multisource queries, the system autonomously selects the most relevant sources, removes irrelevant data and integrates insights cohesively before generating a response.
Context-aware modality handling: RAG dynamically adjusts retrieval strategies based on text, structured data, images, PDFs and other formats.
Trust and grounding mechanisms: Agentic RAG incorporates self-correction, verification and fact-checking for more accurate responses that are well grounded in enterprise data.
Personalization and strategic adaptation: Enterprise users have different needs. Agentic RAG tailors retrieval and generation strategies to optimize for accuracy, latency and cost based on specific business priorities.
Why this matters for enterprise AI
By adding judicious decision-making, iterative refinement and contextual awareness, agentic RAG makes enterprise AI systems far more powerful, efficient and reliable than traditional approaches.
Introducing Arctic Agentic RAG: Bridging research and real-world AI
At Snowflake, we are developing Arctic Agentic RAG — a framework designed to seamlessly integrate agentic intelligence into enterprise AI applications.
Our goal is to bridge the gap between cutting-edge AI research and real-world business use cases by making RAG:
More intelligent: Adaptive reasoning for better query understanding and retrieval.
More scalable: Efficiency, speed, and cost-optimized retrieval and generation for enterprise workloads.
More reliable: Trustworthy, verifiable and explainable AI for high-stakes domains.
In this blog series, we will explore:
The core innovations behind Arctic Agentic RAG, including advancements in algorithms, methodology and the use of data sets, benchmarks and evaluation metrics to measure effectiveness
How Arctic Agentic RAG integrates into Snowflake’s AI-powered products
How enterprises can leverage its capabilities to interact with their data
Our commitment to open sourcing the framework and enabling the broader AI community to build and extend their own agentic RAG applications
A research-driven, iterative approach
AI is evolving rapidly, and so is our research. Rather than presenting a finalized solution, we will share our progress iteratively, tackling one enterprise AI challenge at a time. Each release will focus on a specific challenge, problem formulation or new insight, allowing us to iteratively refine our approaches based on real-world experimentation and feedback. Rather than presenting a finalized solution up front, we aim to share our iterative journey, continuously improving along the way.
Our first challenge? Handling ambiguous queries in enterprise AI.
Check out our Episode 1 blog post. We dive into query ambiguity and how Arctic Agentic RAG addresses it with grounded and speedy responses. We will also provide an overview of the Arctic Agentic RAG open source framework there.
Interested in this discussion? Visit the AI Research & Development Community forum on Snowflake.