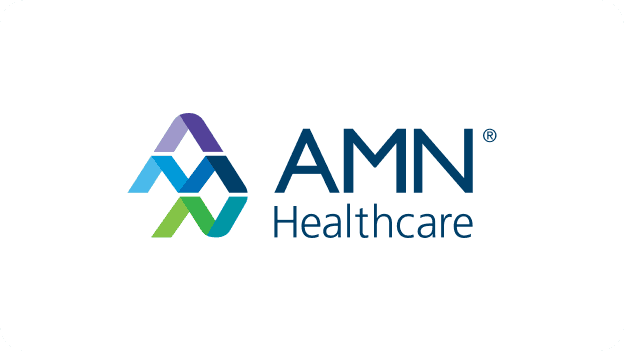
Data lake vs. data warehouse vs. data mart
Explore the unique characteristics and differences between data lakes, data warehouses and data marts, and how they can complement each other within a modern data architecture.
- Overview
- Data Lakes
- Data Warehouses
- Data Marts
- Comparative Overview
- Integrating Data Solutions
- Resources
Overview
In today's data-driven landscape, organizations employ various storage solutions to manage and analyze their data effectively. Among these, data lakes, data warehouses and data marts are prominent, each serving a distinct purpose. This article explores their unique characteristics, differences and how they can complement each other within a modern data architecture.
Data lakes
A data lake is a centralized repository designed to store vast amounts of raw data in its native format, whether structured, semi-structured or unstructured. This approach allows organizations to ingest data from diverse sources without the need for immediate transformation, making it ideal for big data analytics, machine learning and real-time monitoring.
Key characteristics of data lakes:
- Storage of raw data: Store data as-is, enabling flexibility for future processing and analysis
- Schema-on-read: Apply structure when data is read, allowing for dynamic and flexible analysis
- Scalability: Designed to handle large volumes of data, scaling as data grows.
- Cost-effectiveness: Often use affordable storage options, which allows organizations to store very large amounts of data inexpensively
Key characteristics of data lakes:
Data science and machine learning: Providing data scientists with access to raw data for exploratory analysis and model development
Real-time analytics: Supporting applications that require immediate insights from streaming data sources
- Data archiving: Storing historical data that may not need immediate processing but is valuable for future analysis
Data warehouses
A data warehouse is a centralized relational database that stores structured and processed data, optimized so organizations can query and analyze data efficiently for business intelligence. It integrates data from various operational systems, providing a unified view for business intelligence, reporting and decision support.
Key characteristics of data warehouses:
Structured data storage: Cleaned, transformed and organized data into schemas, such as star or Snowflake schemas
Schema-on-write: Defined structure before data is loaded, to help ensure consistency and reliability
High performance: Optimized for complex queries and analytical workloads, often with indexing and partitioning strategies
- Data integration: Data combined from multiple sources, enabling a cohesive dataset for analysis
Use cases for data warehouses:
Business intelligence: Enabling organizations to generate reports and dashboards for strategic decision-making
Historical data analysis: Analyzing trends over time to inform business strategies
- Regulatory compliance: Maintaining structured records to address industry regulations and standards
Data marts
A data mart is a focused subset of a data warehouse, tailored to serve the specific needs of a particular business unit, department or user group. By concentrating on a single subject area, data marts provide streamlined access to relevant data, enhancing performance and user autonomy.
Key characteristics of data marts:
Subject-specific: Designed for specific areas such as sales, finance or marketing
Simplified design: Smaller and less complex than data warehouses, making them easier to manage
Faster access: Optimized for the specific queries and reports needed by the targeted user group
- Autonomy: Allow departments to control their data and tailor solutions to their unique requirements
Use cases for data marts:
Departmental reporting: Providing teams with the data they need without accessing the entire data warehouse
Performance optimization: Reducing the load on the central data warehouse by offloading specific queries
- Cost management: Implementing cost-effective solutions for departments with limited data needs
COMPARATIVE OVERVIEW
Understanding the distinctions between data lakes, data warehouses and data marts is crucial for designing an effective data strategy. The following table summarizes their key differences:
Aspect
Data lake
Data warehouse
Data mart
Data types
Raw, unprocessed (structured, semi-structured, unstructured)
Processed, structured
Processed, structured
Schema
Schema-on-read
Schema-on-write
Schema-on-write
Scope
Enterprise-wide
Enterprise-wide
Department-specific
Size
Large-scale
Large to medium-scale
Smaller-scale
Users
Data scientists, engineers
Business analysts, decision-makers
Specific department users
Purpose
Exploratory analysis, machine learning
Reporting, business intelligence
Targeted analysis, departmental reporting
Integrating data solutions for AI and analytics
While data lakes, data warehouses and data marts each have distinct functions, they can work together effectively as parts of a cohesive data architecture:
Data lake as a foundation: The data lake acts as a central repository for all raw data, capable of handling diverse data types and sources, and providing a strong foundation for AI and machine learning applications.
Data warehouse for structured analysis and AI: The data warehouse processes and structures data from the data lake to enable high-performance analytics and AI, helping ensure data is ready for machine learning algorithms and AI models.
- Data marts for specialized needs and AI applications: Data marts extract pertinent data from the data warehouse to fulfill the specific requirements of individual departments or AI applications, helping ensure that AI models have access to the most relevant data.
This layered approach allows organizations to get the most out of their data, providing flexibility for data scientists to develop AI and machine learning models and robust tools for business analysts to generate insights.
Ultimately, selecting the appropriate data storage solution depends on an organization's specific needs. These include the types of data they handle, the users accessing the data and the intended use cases, including AI and machine learning initiatives. By understanding the unique features and benefits of data lakes, data warehouses and data marts, businesses can design a data architecture that supports both their current requirements and future growth, particularly in the area of AI-driven analytics.