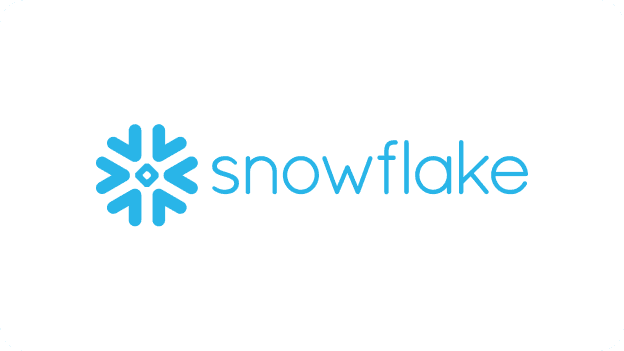
What is synthetic data?
Unlike traditional data, which is often restricted by a range of accessibility issues, synthetic data is artificially generated, providing extensive opportunities for data-driven insights.
- Overview
- What Is Synthetic Data?
- Benefits of Synthetic Data
- How Synthetic Data Is Generated
- Applications of Synthetic Data
- Real-World Examples of Synthetic Data
- Future of Synthetic Data
- Resources
Overview
Synthetic data is revolutionizing how organizations manage and analyze information. Unlike traditional data, which is often restricted by accessibility issues, synthetic data is artificially generated, providing extensive opportunities for testing, AI model training and data-driven insights. This innovative approach allows businesses to experiment and test their models without the limitations of real-world data. In this comprehensive overview, we will explore the definition of synthetic data, its benefits, methods of generation and practical applications. By understanding synthetic data, organizations can unlock new avenues for innovation and enhance their decision-making processes.
What is synthetic data?
Synthetic data is artificially generated information that can approximate the statistical properties of actual data, making it useful for various applications such as machine learning, testing and analytics. Characteristically, synthetic data is devoid of personally identifiable information (PII), ensuring it does not expose sensitive details about real individuals or organizations. It can be customized to meet specific requirements, allowing users to create data sets that reflect different scenarios without the limitations of real-world data.
One of the key distinctions between synthetic data and real data is the ability to control and manipulate the data set. Synthetic data can be produced in large volumes and can include a diverse range of variables, which aids in training algorithms and reduces the risk of overfitting to the nuances found in real data. Additionally, while real data can be biased or incomplete, synthetic data can be designed to mitigate such inconsistencies, providing a more balanced representation for analysis.
The importance of synthetic data in data privacy and security cannot be overstated. By using synthetic data sets, organizations can mitigate privacy risks associated with handling real data. This is particularly crucial in industries like healthcare and finance, where data breaches can have significant repercussions. With synthetic data, organizations can innovate and conduct research without compromising individual privacy, fostering a more secure environment for data use.
Benefits of synthetic data
Synthetic data offers several compelling advantages, especially in training AI models. Here are a few key benefits:
- Increased data availability and data privacy: Traditional methods of data collection can be time-consuming and limited by privacy concerns, making it challenging to gather enough quality data for effective training. Synthetic data, on the other hand, can be generated quickly and in large volumes, enabling data scientists to access the diverse data sets they need without the constraints of real-world data.
- Ability to reduce bias and increase diversity: Real-world data often reflects existing biases, which can lead to skewed AI outcomes. By creating synthetic data sets that intentionally include more variety (for example, different scenarios and demographics), organizations can develop more balanced AI models. This improved diversity helps ensure that AI solutions are fairer and more representative of different groups, ultimately leading to better decision-making and outcomes.
- Cost effectiveness: Acquiring and processing real-world data can be expensive due to data licensing fees, storage costs, and regulatory compliance. Generating synthetic data can reduce many of these expenses, allowing companies to allocate resources more effectively.
How synthetic data is generated
Synthetic data generation creates artificial data rather than collecting it from real-world events. This can be done through various methods, like statistical techniques, rule-based systems or advanced machine learning algorithms. Each method has its own advantages, allowing for the generation of data that looks very similar to real data, but with the added ability to easily change specific details.
Synthetic data generation relies heavily on algorithms and machine learning. These technologies analyze real data sets to learn their patterns and characteristics. Powerful generative models, like generative adversarial networks (GANs) and variational autoencoders (VAEs), play a pivotal role in the process. Using these models, organizations can create large amounts of synthetic data that closely reflects the statistical properties of the original data, making it useful for training machine learning models and performing analyses.
However, generating synthetic data can be challenging. Quality assurance and validation are critical to ensure the synthetic data sets are reliable and useful, incorporating techniques such as:
Statistical testing: Comparing statistical properties, such as distributions, means and standard deviations, between the synthetic and real data sets to ensure fidelity
Visualization comparisons: Using visual representations such as histograms or scatter plots to identify discrepancies and assess how well the synthetic data mirrors the real data's patterns
- Domain-specific assessments: Applying validation criteria specific to the intended use case — for example, ensuring synthetic patient records adhere to medical data standards
These validation processes are essential for establishing trust in synthetic data sets, enabling organizations to confidently leverage them for informed decision-making and robust model training, and ultimately enhancing the efficacy of data-driven initiatives.
Applications of synthetic data
Synthetic data is transforming industries by providing innovative solutions across various industries. Here are some examples:
In healthcare, synthetic data can be generated to create realistic patient records that facilitate research while providing anonymization and aggregation. This enables medical researchers to develop and test algorithms for diagnostics and treatment while adhering to strict data protection regulations.
In finance, synthetic data plays a crucial role in risk assessment and fraud detection. Financial institutions can generate diverse data sets to simulate market conditions and customer behaviors, helping them to refine their models and improve decision-making processes. This accelerates the development of financial technologies and enhances the security of financial transactions.
In manufacturing, auto companies can use synthetic data to simulate a myriad of driving scenarios for autonomous cars. They can then train machine learning models to recognize and respond to various conditions without the need for extensive real-world data collection. This not only speeds up the testing process but also ensures that vehicles are safer and more reliable.
Across sectors, the use of synthetic data significantly impacts research and development by enabling companies to innovate while reducing the risks associated with handling sensitive information. By creating data sets that mimic real-world scenarios, businesses can explore new ideas and solutions to drive innovation without the fear of breaching compliance regulations.
Real-world examples of synthetic data
Future of synthetic data
The future of synthetic data is marked by a convergence of powerful trends. Advancements in generative AI are enabling the creation of increasingly realistic and complex synthetic data sets, blurring the lines between artificial and real data. Simultaneously, the growing regulation-driven focus on data privacy and security is pushing organizations to explore synthetic data as a viable alternative to using sensitive real-world information. This confluence of technological capability and regulatory pressure is creating a fertile ground for synthetic data adoption across diverse industries in the near future.
However, the rise of synthetic data is not without its challenges and ethical considerations. As the technology becomes more sophisticated, questions arise regarding the authenticity and trustworthiness of synthetic data sets. Ensuring transparency in how synthetic data is generated and used will be essential to address concerns about data misuse and the potential for reinforcing biases. Moreover, regulatory frameworks will need to adapt to this evolving landscape to safeguard ethical standards in data usage.
With careful attention to ethical considerations and robust validation practices, synthetic data has the potential to revolutionize how we use and interact with data, driving progress in fields ranging from drug discovery to personalized finance.